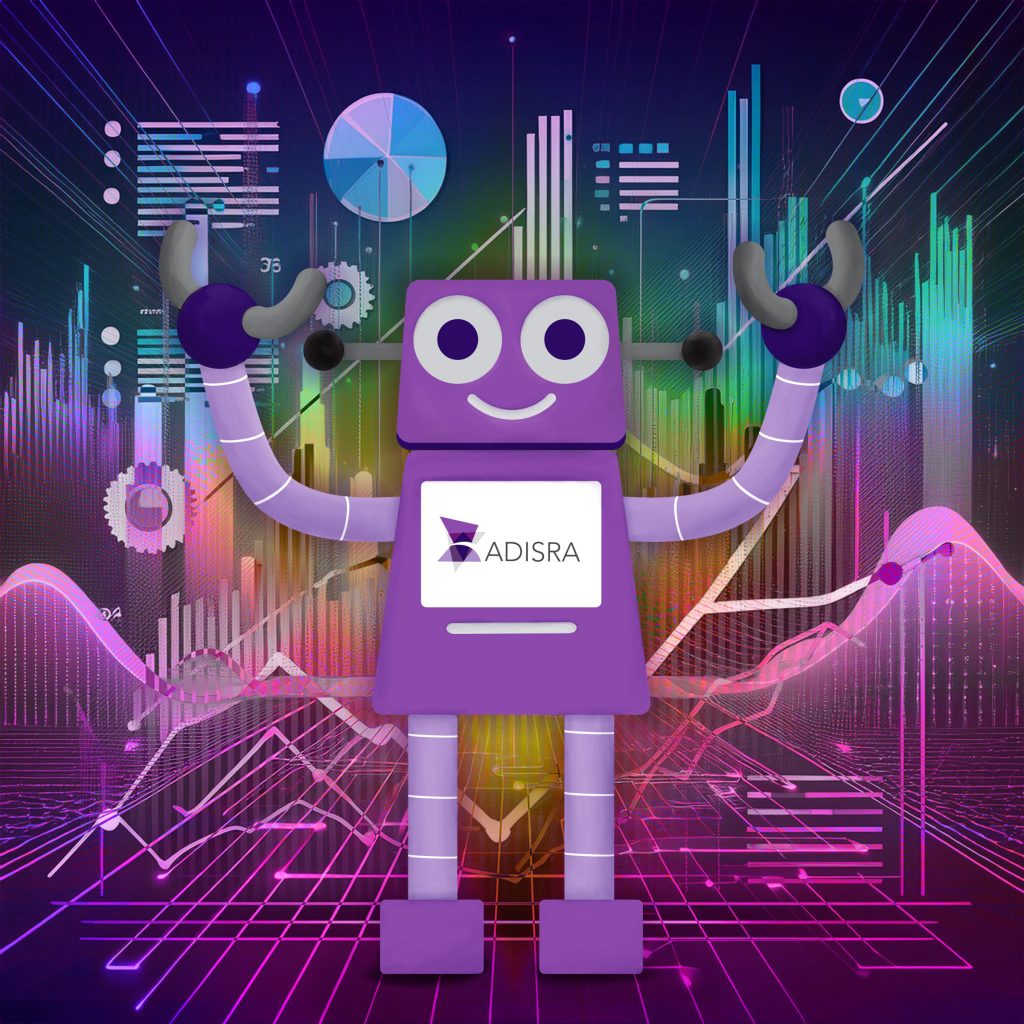
Decision-makers are increasingly adopting edge analytics to optimize processes and boost operational efficiency in today’s fast-paced industrial landscape. Manufacturers can process real-time data directly at the source—whether on the factory floor or in the field—by integrating HMI/SCADA systems with advanced analytics. According to ARC’s Industrial Edge Computing Market report, the industrial edge computing market is projected to grow to $11.5 billion by 2030, with a compound annual growth rate (CAGR) of 12.5% between 2024 and 2030. This transition to edge analytics enables quicker, data-driven insights while reducing latency, bandwidth usage, and reliance on cloud infrastructure.
What Is Edge Analytics in Industrial Automation?
Edge analytics refers to analyzing data and finding solutions at the edge, where data is generated—such as sensors, machines, or industrial equipment—rather than sending it to a centralized cloud or data center. This enables real-time monitoring and analysis, which is essential for reducing downtime, optimizing performance, and making quick decisions.
The Path to Analytics Transformation.
The data analytics journey in industrial automation consists of five phases, beginning with descriptive analytics and advancing to cognitive analytics. Each phase brings the organization closer to full data maturity, enhancing its ability to make strategic, informed decisions that improve operational efficiency and competitiveness.
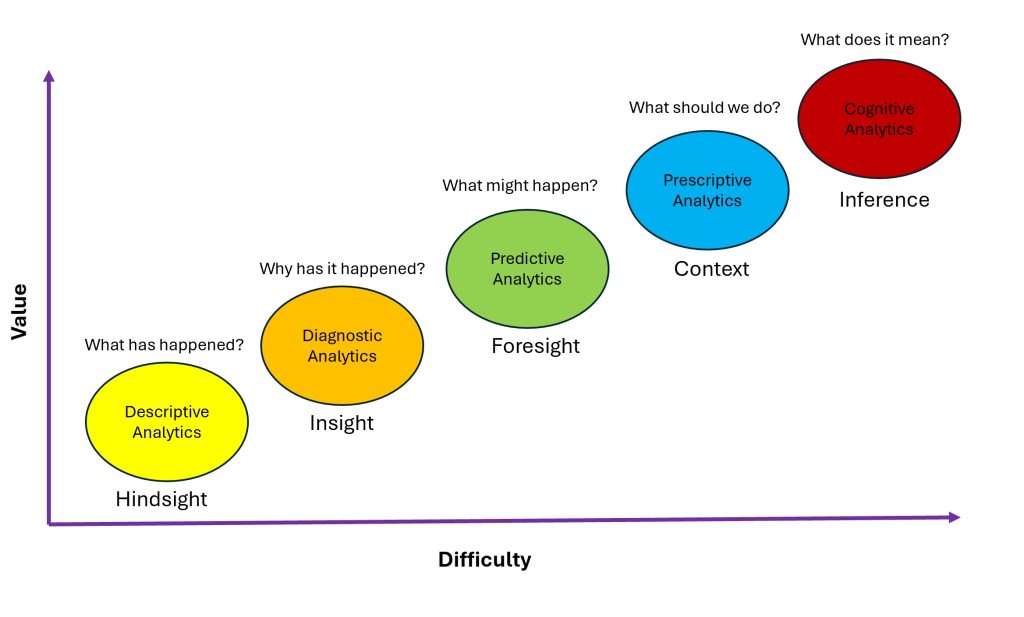
Descriptive Analytics
Descriptive analysis is one of several methods used to gather and structure raw data and derive meaningful insights.
Descriptive analytics specifically focuses on summarizing and interpreting historical data to understand past events, patterns, and trends clearly. It answers the question, “What has happened?” Using various statistical tools and techniques, descriptive analytics explores data to uncover insights into its characteristics, behaviors, and relationships.
Descriptive analytics might involve tracking machine performance on a factory floor using historical data. For example, a manufacturer may monitor key performance indicators (KPIs) like production output, machine downtime, and energy consumption over time. This data is then presented in reports or dashboards, allowing operators and managers to understand past performance, identify patterns, and detect inefficiencies, such as extended downtime or reduced productivity. Descriptive analytics offers a comprehensive view of past operations, enabling timely adjustments to optimize performance.
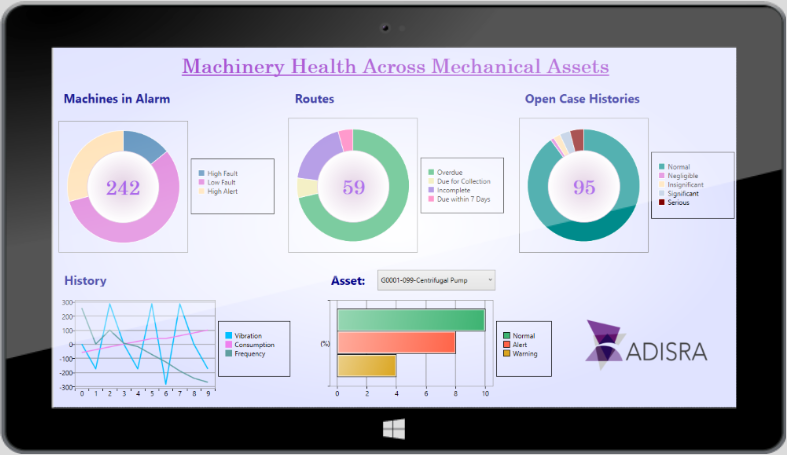
With a rule-based expert system, you can create rules that automatically interpret data. For instance, you could set rules to detect if production output drops below a threshold or if a machine’s energy consumption exceeds a standard level. These rules can trigger alerts or actions based on the summarized data.
Suppose a factory uses an HMI/SCADA system with a rule-based expert system to monitor production line efficiency. You can log the machine’s operational data, such as output rates and energy consumption. The rule-based system is programmed to automatically generate summaries when specific thresholds are crossed (e.g., when output drops below a target level). This descriptive analysis can be displayed in real-time dashboards, allowing operators to identify performance trends over a period. If the analysis shows periods of low productivity, further diagnostic analysis can be triggered to identify root causes.
Diagnostic Analytics
If descriptive analytics focuses on what happened, then diagnostic analytics seeks to explain why it happened. Diagnostic analysis dives deeper into data to uncover specific events’ underlying reasons and root causes. Its primary goal is to understand the factors contributing to past outcomes, answering the question, “Why has it happened?” or “Why did this occur?” By examining relevant variables, diagnostic analytics helps identify what influences certain results.
For example, diagnostic analytics can be used to determine the cause of unexpected machine downtime. Imagine a production line experiencing frequent downtime over the past few months. The system can analyze various operational factors during downtime, such as machine settings, environmental conditions, maintenance schedules, operator shifts, and sensor data by applying diagnostic analytics.
The analysis might reveal that the downtime often coincided with a specific machine component reaching a certain temperature threshold or when the equipment was run under particular load conditions. This insight allows operators to identify the issue’s root causes and take corrective action to prevent future occurrences.
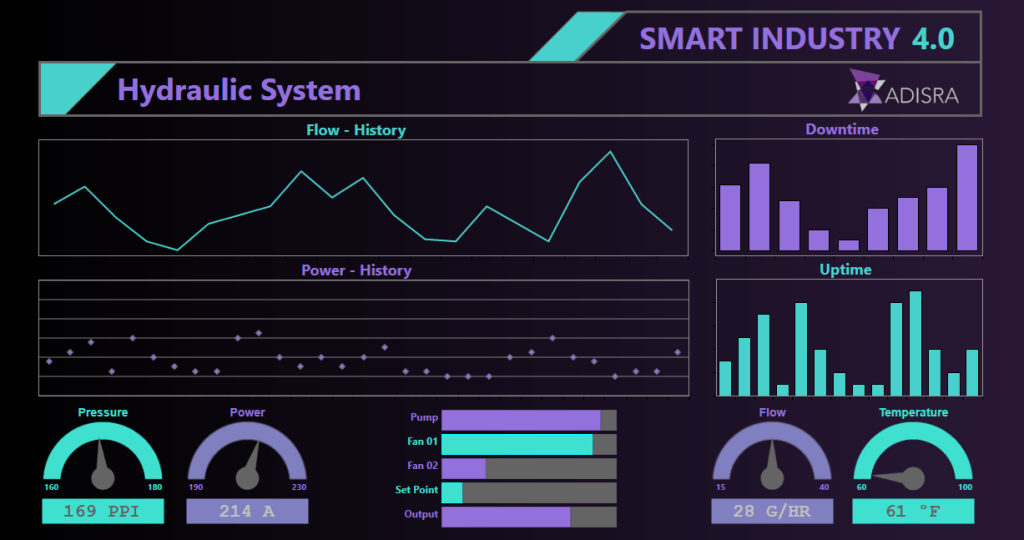
Predictive Analytics
Predictive analytics focuses on forecasting future events by analyzing historical data. These forecasts can vary from short-term predictions, like anticipating a machine’s malfunction later in the day, to long-term projections, such as foreseeing machinery supply shortages for the next year. By recognizing patterns and trends in the data, predictive analytics helps answer the critical question: “What might happen?”
A key application of predictive analytics in industrial automation is predictive maintenance. HMI/SCADA systems continuously gather data from machine sensors, monitoring factors like temperature, vibration, pressure, and operational speed. Predictive analytics processes this data to detect patterns that suggest potential equipment failures. For instance, if vibration levels in a machine steadily increase, the system can predict a likely bearing failure. In this case, a rule-based expert system can automatically trigger a maintenance alert, prompting operators to inspect or replace the bearing before it causes unplanned downtime or disrupts production. This proactive approach minimizes unexpected equipment failures and optimizes the maintenance schedule.
For example, a facility might use an HMI/SCADA system integrated with a rule-based expert system to monitor critical production pumps. These pumps are outfitted with sensors that continuously measure parameters such as:
– Vibration levels
– Temperature
– Pressure
– Flow rates
The rule-based expert system is preconfigured with rules based on operational thresholds and known pump failure patterns. These rules detect abnormal conditions and initiate actions to prevent failures or downtime.
As the pumps operate, the HMI/SCADA system monitors the data in real-time. When one of the predefined rules is triggered, such as an increase in vibration or temperature, the rule-based expert system acts automatically according to the programmed logic. This might include generating alerts, logging incidents, or taking corrective action, like shutting down equipment to prevent damage.
By continuously monitoring these parameters and applying the expert system’s rules, potential issues are detected early. This allows maintenance teams to intervene before pump failures occur, reducing unplanned downtime and improving equipment reliability.
Prescriptive Analytics
Prescriptive analytics, in contrast, answers the question “What should we do?” by determining the optimal course of action based on available data. This process considers all relevant factors and provides recommendations for next steps. Machine-learning algorithms are often employed in prescriptive analytics, analyzing real-time data and applying “if-else” logic to generate recommendations based on specific conditions.
Prescriptive analytics leverages data, algorithms, and rule-based expert systems to predict potential issues and recommend the best actions to resolve or prevent them in industrial automation. For example, suppose an HMI/SCADA system detects abnormal sensor readings, such as rising temperatures or increasing vibrations. In that case, prescriptive analytics can suggest specific maintenance actions or operational changes to address the problem. It might recommend optimal times for maintenance, adjust operational settings to extend equipment life, or even automate corrective actions like reconfiguring system parameters or scheduling a maintenance task. This approach minimizes downtime, optimizes resource allocation, and improves operational efficiency by transforming data insights into actionable recommendations.
Cognitive Analytics
Much of the data in industrial automation exists in spreadsheets, notes, and other unstructured formats, making it difficult to collect and analyze effectively. This is where cognitive analytics comes into play, helping to answer the question: “What does it mean?”
Cognitive analytics is a cutting-edge form of data analysis that leverages AI and machine learning to process large volumes of unstructured data. Using advanced AI technologies, such as natural language processing (NLP) and deep learning algorithms, cognitive analytics can extract valuable insights and generate predictions from this complex information, turning raw data into meaningful intelligence for decision-making.
Analytics on the Edge focuses on transitioning from descriptive to prescriptive analytics, enabling real-time decision-making directly at the data source. More advanced processes, such as cognitive analytics, are typically reserved for centralized big data systems, where vast amounts of unstructured data can be processed using sophisticated AI techniques. This division allows immediate operational insights and actions to be managed locally, while more complex data analysis is handled by dedicated systems.
A rule-based expert system integrated within an HMI/SCADA solution further enhances edge decision-making by applying predefined rules to the collected data. These rules, derived from years of industry expertise, best practices, and regulatory standards, enable the system to automatically trigger alarms, initiate corrective actions, or schedule preventive maintenance based on real-time conditions. This type of system can be applied across descriptive, diagnostic, predictive, and prescriptive analytics, streamlining operations and ensuring optimal performance by swiftly responding to evolving scenarios.
What are the advantages of Analytics on the Edge?
Analytics on the Edge provides significant advantages by processing data locally at the source instead of relying entirely on centralized systems. Key benefits include:
Reduced Latency
Processing data at the edge eliminates delays associated with transmitting data to the cloud or a remote data center, enabling critical decisions to be made in milliseconds. For example, monitoring and controlling robotic arms on an assembly line requires minimal latency in an industrial automation HMI application. These robotic arms work at high speeds, and precise control is essential to maintaining production efficiency and preventing defects. Sensors continuously gather data on temperature, vibration, and motor speed. If this data had to be sent to a cloud system for analysis, the resulting delay (latency) could lead to equipment malfunctions, product defects, or even safety risks. Edge analytics ensures real-time decisions are made directly at the source, avoiding such issues.
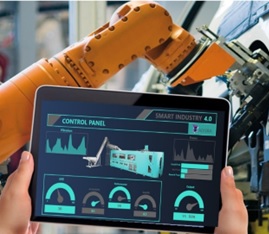
Improved Reliability
Decentralizing data processing allows industrial systems to continue operating even during network disruptions or cloud outages, enhancing overall system resilience.
Bandwidth Efficiency
By sending only relevant or summarized data to the cloud, businesses can reduce bandwidth costs and lessen the strain on centralized systems.
Real-Time Decision-Making
With real-time insights processed locally, operators can respond quickly, increasing the agility and responsiveness of industrial processes.
The Role of HMI/SCADA in Edge Analytics
Human-machine interface (HMI) and Supervisory Control and Data Acquisition (SCADA) systems are essential for collecting and visualizing data from industrial operations. When integrated with edge computing, these systems become even more powerful, improving operational efficiency, reliability, and decision-making by processing data directly at the source. ADISRA SmartView, equipped with a built-in rule-based expert system, offers users a straightforward and intuitive way to embark on their analytics journey. With its user-friendly interface, users can focus on defining rules and achieving desired outcomes. To test ADISRA SmartView, please use the link here.
Conclusion
Implementing edge analytics in industrial automation offers transformative benefits by enabling real-time decision-making, improving system reliability, and optimizing bandwidth usage. By processing data directly at the source, organizations can significantly reduce latency, critical for maintaining the precision and speed required in applications like HMI/SCADA systems. This approach ensures greater operational efficiency and boosts resilience by keeping processes running smoothly even during network interruptions. As industries evolve, leveraging analytics on the edge will be key to staying competitive and responsive in increasingly complex environments.