KnowledgeView: Illuminating Tomorrow's Maintenance Needs Today
Advanced Predictive Analytics for Informed Decision-Making
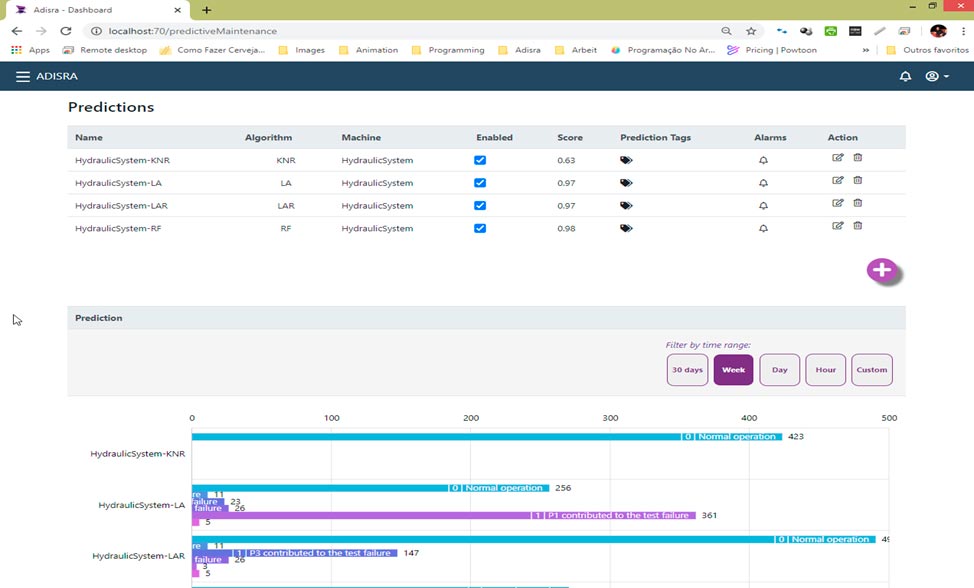
Data transformed into Knowledge
In the dynamic realm of today’s industrial landscape, where staying competitive hinges on maximizing operational efficiency and minimizing downtime, the spotlight turns to predictive maintenance software applications. An innovative approach that offers organizations a unique method for asset maintenance by accurately predicting and addressing future failures.
By leveraging algorithms and machine learning, predictive maintenance anticipates potential equipment or machinery failures. KnowledgeView® specializes in predictive modeling for equipment and process behavior, providing insights into the query, “What potential outcomes could arise?” Additionally, KnowledgeView incorporates prescriptive analytics to address the question, “What actions are recommended or should be taken?”
KnowledgeView
KnowledgeView is designed to cater to customers’ requirements in the small-to-mid-market segment. Operating on a software-as-a-service model mandates adherence to a structured procedure for constructing efficient models tailored to the needs of our target customers.
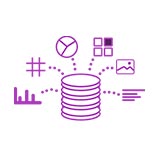
Data Collection:
KnowledgeView begins with the collection of relevant data from the equipment being monitored. This data can include sensor readings, performance metrics, temperature, vibration, and other relevant parameters. The more comprehensive and high-quality the data, the more accurate the predictive models can be.
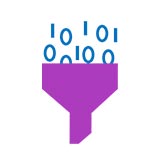
Data Cleansing:
Data cleansing involves rectifying or eliminating inaccurate, corrupted, improperly formatted, duplicated, or incomplete data within a dataset. Integrating data from multiple sources increases the likelihood of duplications or mislabeling. Inaccurate data compromises the reliability of outcomes and algorithms. It's important to note that this stage is focused on improving data quality rather than introducing bias.
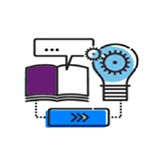
Data Modeling:
Machine learning algorithms, such as regression, decision trees, random forests, or more advanced techniques like neural networks, are then employed to train predictive models. These models learn patterns and relationships within the historical data, associating specific patterns with impending failures.
What is KnowledgeView?
ADISRA KnowledgeView empowers users with understanding of asset risk, enabling them to uphold elevated levels of asset availability across machines, processes, or organizations. Operating on a software-as-a-service model, KnowledgeView utilizes a range of predictive analytics to analyze extensive datasets, identifying potential issues and predicting downtime, outages, and failures before they materialize. The platform supports structuring assets into hierarchies, whether based on machines, production lines, plants, or multiple facilities. This capability enhances operational insights and facilitates strategic maintenance planning.
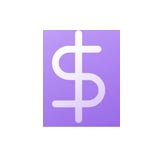
Cost Savings:
Predictive maintenance minimizes unnecessary preventive maintenance, reducing overall maintenance costs.
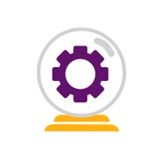
Optimized Resource Allocation:
Maintenance resources can be allocated more efficiently based on predictive insights, avoiding unnecessary interventions.
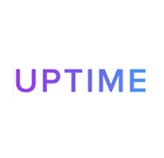
Increased Uptime:
By predicting failures before they occur, equipment downtime is minimized, contributing to higher operational availability.
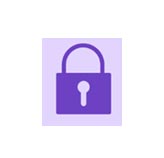
Enhanced Safety:
Early detection of potential failures improves workplace safety by preventing accidents related to equipment malfunctions.
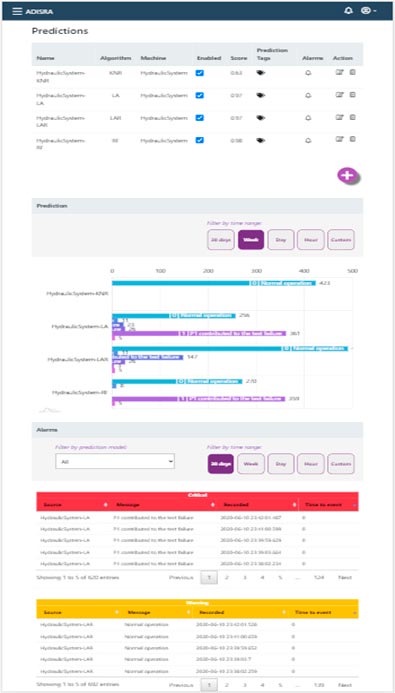
ADISRA KnowledgeView stands as a comprehensive and forward-thinking solution in the realm of predictive maintenance. Operating on a software-as-a-service model, its diverse predictive analytics sift through extensive datasets, proactively identifying and predicting potential issues such as downtime, outages, and failures. By providing users with comprehensive insights into asset risk, KnowledgeView ensures higher levels of asset availability and enhanced overall operational resilience.